Researchers from Nextmol, in collaboration with L'Oréal SA, have published the paper “Predicting the Glass Transition Temperature of Biopolymers via High-Throughput Molecular Dynamics Simulations and Machine Learning” in the journal ACS Applied Polymer Materials.
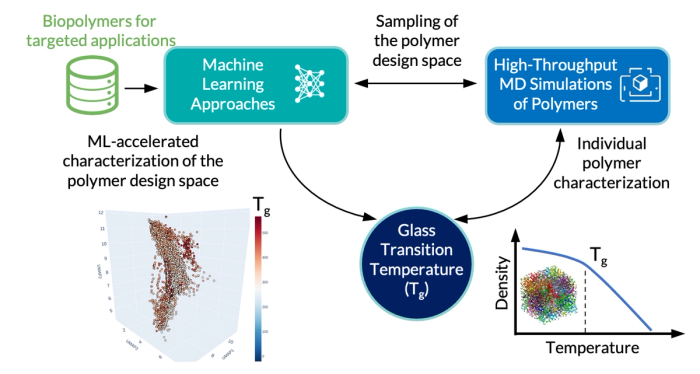
The paper shows that the combination of machine learning (ML) approaches and high-throughput molecular dynamics (MD) simulations permits quick and efficient sampling and characterization of the relevant chemical design space for specific applications, such as biopolymers. Reliable simulation protocols have been implemented to evaluate the glass transition temperature (Tg) of a series of 58 homopolymers and 488 copolymers. These results, constituting a data set of 546 polymers, have been used to train a ML model for the prediction of the MD-calculated Tg with a mean absolute error of 19.34 K and an R2 score of 0.83.
Overall, within its applicability domain, this machine learning model provides an impressive acceleration over molecular dynamics simulations: the Tg of thousands of polymers can be obtained within seconds. This type of approach has the potential to accelerate the discovery of polymers.
The ML model for the prediction of Tg can be accessed here: https://biopolymer-ml-pub.nextmol.com/
Reference: Predicting the Glass Transition Temperature of Biopolymers via High-Throughput Molecular Dynamics Simulations and Machine Learning Didac Martí, Rémi Pétuya, Emanuele Bosoni, Anne-Claude Dublanchet, Stephan Mohr, and Fabien Léonforte ACS Applied Polymer Materials (2024); DOI: 10.1021/acsapm.3c03040
Pre-print available here.
For more information, read the full article or contact us.