Under appropriate pressure and temperature conditions, the self-assembly of water molecules can result in the formation of gas hydrates, a solid, ice-like material that is composed of molecules of appropriate size (typically small hydrocarbon species) enclathrated in three-dimensional water cages. Due to the favorable conditions, gas hydrates can form spontaneously during oil and gas production and threaten the safety and economic viability of the operation processes. Indeed, as oilfields mature, they produce increasing quantities of water that can lead to hydrate nucleation, growth and agglomeration, and potentially cause pipeline blockage.
There exist several ways to mitigate this problem: Either via thermodynamic inhibitors, which ensure that the temperature and pressure conditions that favor hydrate formation lie outside of the operation range, or via low-dosage inhibitors, which either delay hydrate nucleation (“kinetic inhibitors”) or prevent the agglomeration of small hydrate particles into larger blockages (“anti-agglomerants (AAs)”). These AAs are typically amphiphilic surfactants containing a polar headgroup and a nonpolar tail.
Since 2019, Nextmol has been working on computational solutions to accelerate the development of new AAs. To this end, we exploit various computational techniques, amongst others Machine Learning (ML) and Molecular Dynamics (MD), to study the fundamental properties of the AAs, their interaction with the hydrate surface and how they can inhibit the agglomeration of hydrate particles, and perform High-Throughput screenings to identify new molecules with enhanced properties.
A particular characteristic of a hydrate crystal is that even below the melting point its surface is covered by a small film of liquid water (a phenomenon called “premelting”), whose thickness depends on the subcooling (i.e. the temperature gap with respect to the melting point). In the context of flow assurance, this liquid layer plays an important role in the interaction between hydrate particles and pipe walls or between hydrate particles themselves by enabling the formation of a capillary bridge, which is one of the dominant mechanisms of the hydrate agglomeration process. However, despite this importance, the liquid layer is often not included in the computational models or it is set up quite arbitrarily.
The shed light upon the behavior of this liquid layer and how it affects the performance of AAs, researches from Nextmol, in collaboration with our client Clariant Oil Services and Clariant Produkte (Deutschland) GmbH, have performed MD simulations to study the adsorption of 10 AAs onto a mixed methane–propane sII hydrate surface covered by layers of liquid water of various thickness. As a general trend, it was found that the more liquid water is present on the hydrate surface, the less favorable the adsorption becomes. However, there are considerable differences between the individual molecules, indicating that the presence and thickness of this liquid water layer are crucial parameters for AA adsorption studies.
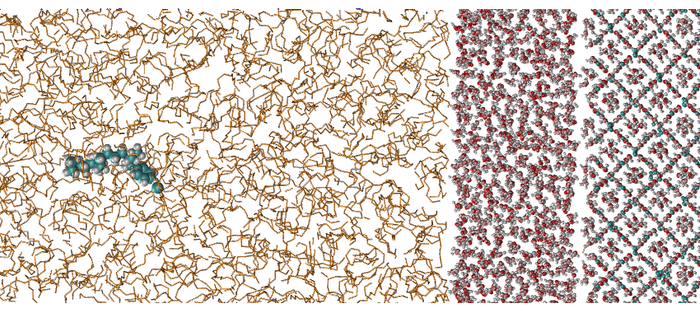
Additionally, we found that there exists an optimal thickness of the liquid water layer favoring hydrate growth due to the presence of both liquid water and hydrate-forming guest molecules. For all other cases of liquid water layer thickness, hydrate growth is slower due to the limited availability of hydrate-forming guests close to the hydrate formation front. Finally, we investigated the connection between the thickness of the liquid water layer and the degree of subcooling and found a very good agreement between our MD simulations and theoretical predictions and experimental measurements.
To summarize, our study indicates that neglecting the liquid layer represents a severe bias and can lead to wrong conclusions. A careful determination of the liquid water layer under the given conditions and its integration into the simulation setup is, therefore, highly advisable. Our study gives indications on how the liquid layer should be set up and, consequently, represents an important reference for future studies concerning simulations of gas hydrates.
All of these valuable insights could be obtained thanks to the atomistic resolution of our MD approach that allows to easily set up any model of interest and model it under and the thermodynamic conditions of interest. Moreover, the execution of the overall 70 simulation setups was facilitated via a High-Throughput approach that allows to execute a large number of simulations in an automated way and with only minimal human interaction. With a purely experimental approach, such a systematic investigation would hardly be possible and, in any case, be very time consuming and costly. This underlies the added value that Computational Chemistry can bring to R&D activities.
This work has been published in The Journal of Chemical Physics. Contact us for more information.